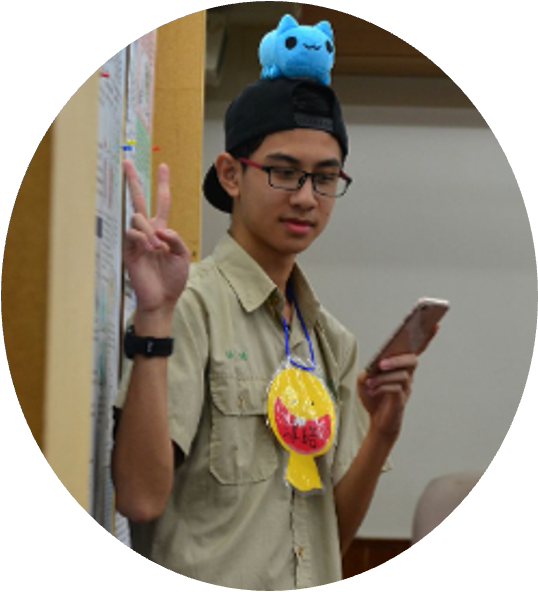
Chris Chang (Research Assistant in ATS)
Chris is a research assistant working on the application of linear stochastic theory to MJO dynamics.
Our Lab focuses on the predictability and future projections of climate extremes from a dynamical system perspective. Using hierarchical modeling framework, data-driven method and statistical mechanics, we study the geophysical fluid dynamics across different temporal and spatial scales, such as tropical intraseasonal variability, subtropical high, baroclinic waves/annular mode, and idealized 2D/3D turbulence. Detailed information can be found in “Publications”.
2023/11 Yu-Chuan Kan undergraduate proposal was funded by NSTC
2023/09/05 Yu-Chuan Kan wins 2nd place in student poster competition of NTU AS summer program
2023/06/15 Dr. Kai-Chih Tseng is awarded 2030 Cross Generation Young Scholar Program by NSTC
2022/08/01 Dr. Kai-Chih Tseng is awarded Yushang Young Scholar by MOE.
Chris is a research assistant working on the application of linear stochastic theory to MJO dynamics.
Yun-Hsuan is a research assistant working on the prediciton and predictability of subtropical high from subseasonal to seasonal timescales.
Jin-De is a research assistant working on the dynamical behavior of annular mode including its persistence/propagation and predictability.
Benjomin Loi is a research assistant working on the information theory and the predictability of tropical cyclones
Shih-Pei Hsu is a Ph.D. student working on the future projection of tropical waves using quasi-equilibrium framework.
Jin-Yu Chang is a 2nd-year M.S. student working on moist baroclinic wave and annular mode dynamics (using GFDL DyCore) and the related predictability.
Hsuan-Cheng Lin is a 2nd-year M.S. student working on monsoon depression/onset with model hierarchy and machine learning.
Ray Kuo is an 1st-year M.S. student working on the information theory and predictability of dynamical systems.
Yi-Hui Huang is an undergraduate student studying the predictability components of subseasonal variability.
Yi-Ann Feng is an undergraduate student studying the numerical solution of 2D and 3D turbulence predictability
Yu-Chuan Kan is an undergraduate student studying the interaction between cumulus/cloud radiative feedback and tropical convectively coupled waves
Weather is intrinsiclly unpredictable due to its chaotic nature. Thus, using a probability density function (PDF) to describe the time evolution of forecast error is central to the prediction problem. However, generating enough ensemble members to approximate the entire PDF can be computationally expensive and unaffordable due to limited window of time for decision making. In this study, we propose a proof-of-concept of generating infinity ensemble members by combing machine learning and statistical mechanics.
The theory for explaning the backbone dynamics of Madden-Julian oscillation has been long pursuit of the scientific community. However, how the existing framework links to the prediction and predictability of MJO is still missing. Here, we provide framework to bridge such gap.
While weather forecast is a high-dimension chaos problem, the statisitcal behavior is closely tied to the idealized 2D/3D turbulence. The characteristics of unlimited/limited predictability of 2D/3D turbulence can help the optimization of computational cost of numerical weather forecast.
The over-persistent bias of annular mode in GCM has been well recognized by the community. The theory responsible for such bias is however less completed. In our group, we use a hierarchical modeling framework to investigate the most fundamental mechanisms.
The Wester North Pacific Subtropical High is playing a critical role in modulating the East Asia hydrological cycle such as the route of tropical cyclone and the location of Meiyu front. It's predictability is however limited on subseasonal to seasonal timescales. Our group use Causal Discovery methods and dynamical system analysis to study its intrinsic predictability limits and the dynamics responsible for such time horizon.
Weng et al., Approaching riverine roles of flying rivers (Submitted to Science Advances)
Chang C.-Y.,(*), K.-C. Tseng, and Y.-C. Kan: Moisture and Persistence of Annular Mode: The Role of Large-Scale Tropical Variability (in preparation)
Hsu, S.-P.,(*) H.-J. Tai, C.-H. Sui, Y.-C. Kan(*), and K.-C. Tseng: The Roles of Shallow Convection and Cloud-Radiative Feedback in Convectively-Coupled Kelvin Waves (in preparation for submission)
Xiao, H.-M., K.-C. Tseng, J.-Y. Yu, H.-H. Hsu, T.-H. Lee, M.-H. Lo,: The Enhanced Tele- connection of Maritime Continent Deforestation on North Pacific Climate During La Nina Conditions (submitted to Journal of Climate)
Tseng K.-C., Ray Kuo(*) and Yi-An Feng(*): Solving the Analytical Solution of Ensemble Forecast with Data-driven Liouville equation (submitted to JAMES)
Tseng K.-C., and co-authors: Skillful forecasts of springtime CONUS tornado activity up to a year in advance (in preparation)
Loi B(*)., K.-C. Tseng and C.-C. Wu: Predictability of Tropical Cyclone Track Density in S2S Reforecast npj Clim Atmos Sci (co-leading) 8(1)24
Schmitt, J.,(*) Tseng, K.-C, Hughes M., and Johnson, N., : Illuminating snow droughts: The future of Western United States snowpack in the SPEAR large ensemble. J. Geophys. Res. 129, e2023JD039754
Tseng K.-C., and Y.-H., Ho(*): The Subseasoanl Predictability of North Pacific Subtropical High and the 2020 Record-breaking Event Npj Clim. Atmos. Sci. 6, 1-9
Zhang, W., Xiang, B., Tseng, K.-C, Johnson, N., Harris L., Delworth T.: Subseasonal prediction of wintertime atmospheric rivers in the GFDL SPEAR model. (accepted by npj Climate and Atmospheric Science)
Bower, C., and coauthors: Atmospheric River Sequences as Indicators of Hydrologic Hazard in Present and Future Climates (submitted to Earth Future)
Jong, B.-T., and coauthors: Investigating Observed and Projected Increases in Extreme Precipitation over the Northeast United States using High-resolution Climate Model Simulations npj Clim Atmos Sci 6(18)
Tseng K.-C., and co-authors: When will humanity notice its influences on atmospheric rivers?127 e2021JD036044. link
Jia L.,and co-authors: Skillful seasonal prediction of North American summertime hot extremesJ. Clim., 35(13), 4331--4345 link (Nature Research highligh)
Bushuk B., and co-authors: Mechanisms of Regional Artic Sea Ice Predictability in Dynamical Seasonal Forecast Systems 35(13), 4207--4231 link
Chen Y.-L.,and co-authors: Effect of the MJO on East Asian winter rainfall as revealed by a SVD analysis J. Clim., 34(25), 9729--9746 link
Zhang L.,and co-authors: Using large ensembles to elucidate the possible roles of Southern Ocean meridional overturning circulation in the Southern Ocean 36-yr SST trend (J. Clim, in press)
Tseng K.-C., and co-authors: Are multiseasonal forecasts of atmospheric rivers possible? Geophys. Res. Lett 48 e2021GL094000. link (NOAA-GFDL and Princeton CIMES Research highligh)
Bushuk B., and co-authors: Seasonal prediction and predictability of regional Antarctic sea ice J. Clim., 34(15), 6207--6233 link
Zhang G., and co-authors: Seasonal Predictability of Baroclinic Wave Activity npj Clim Atmos Sci 4(50) link
Tseng K.-C., N. C. Johnson., E. D. Maloney, E. A. Barnes, and S. B. Kapnick: Mapping Large-scale Climate Variability to Hydrological Extremes: An Application of the Linear Inverse Model to Subseasonal-to-Seasonal prediction J. Clim., 34(11), 4207--4225 link
Tseng K.-C., E. A. Barnes, and E. D. Maloney: The important role of the MJO for extratropical variability in observations and the CMIP5 climate models (submitted to JGR-Atmosphere)